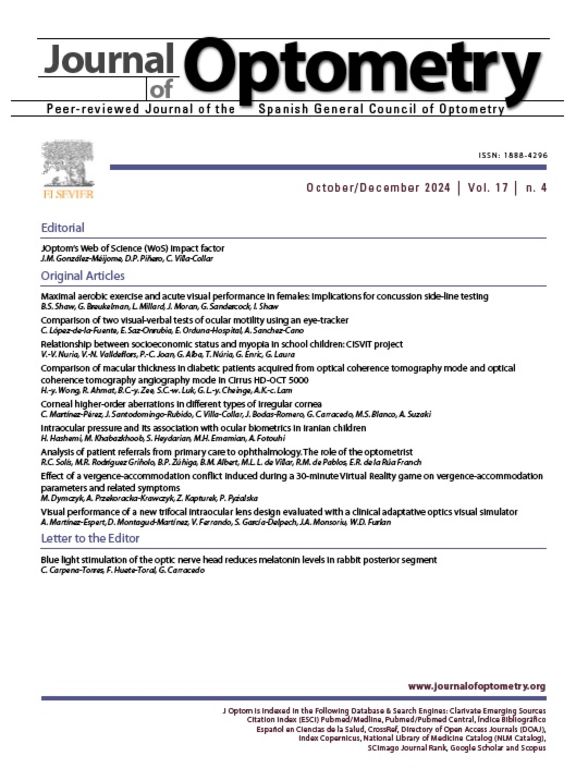
Artificial Intelligence, Data Science and E-health in Vision Research and Clinical Activity
More infoDigital health technology is increasingly becoming part of the evolution of health services, not only for the innovation of equipment but also in support of health processes. Eye health is one of the areas that most explores this field, being a reference in different segments of digital health and the use of applied technological resources. Thus, the purpose of this review was to analyse and characterize the development of research in digital health applied to vision sciences in the last decade. An exploratory-quantitative review of the research based on studies indexed in the SCOPUS database in the last 10 years, which related aspects of digital health technologies with their use within the vision sciences, was conducted. The research results were filtered, including journal articles and excluding those not directly related to vision. The final sample was categorized and classified according to the technology used, the relationship with eye/visual health and its practical applications. A total of 1069 reports were identified (32.09% published since 2021). “Artificial Intelligence” (77.74%) was the most frequent technological tool cited, and posterior segment (68.10%) most eye structure studied, being diabetic retinopathy (27.88%) the main studied disease. The vast majority have potential for clinical use (93.73%), especially those aimed at supporting decision-making. Technologies in digital health in the vision sciences have had a huge growth in recent years, with emphasis on artificial intelligence applied to the posterior segment, but with a low development of studies aimed at using this technology in primary visual care.
Technology applied to health is increasingly becoming a fundamental part of the development of this sector, not only through innovation through specialist equipment but also in supporting different processes and steps related to health care.1 Thus, different technological advances, such as the growth in the use of applications for smartphones, artificial intelligence algorithms, data storage in the clouds, among others, in addition to changes in the population's habits, have been directly reflected in a readaptation of health services.2,3 The applied use of different technologies of “digital health” has attracted great interest in recent years and was amplified by the COVID-19 pandemic4,5 representing an ecosystem that encompasses various ramifications within the health area,6 being recognized in the world of entrepreneurship as “Health techs”.5
Elementary concepts in digital healthThe constant evolution of the technological process sometimes makes it difficult to define definitive concepts for some terminologies, while taxonomies are constantly being proposed or readjusted according to new understandings of the processes involved. Therefore, a current definition of widely used terms is provided to update and guarantee the understandability of this report.7
eHealth/mHealth: The term eHealth refers to the use of different information and communication technologies (ICT) to support any health service. Its scope is directly related to a wide range of applications (generally based on web/app) and different technologies with different purposes that propose to improve, speed up, customize or simplify the processes related to health care.8 Within this area, the concept of mHealth is usually used to explain a subarea of eHealth, characterized to propose solutions exclusively based on mobile applications.9
Telehealth/Telemedicine: eHealth systems can have different purposes, among which the most prominent are telehealth applications. That is, any means that facilitates and/or enables interactions at a physical distance between the patient and access to health can be considered a telehealth service. Although the term telehealth is more generic to all types of health services, the terminology of telemedicine is commonly used to equally designate this health service model.10 It is noteworthy that telemedicine services, although studied for a long time, gained special prominence and relevance from the need for social distancing caused by the pandemic, a factor that contributed to the already growing development of this market.11,12
Artificial intelligence (AI): The concepts of AI were not originally associated with the health area because this term was defined in the 1950s in computer science, but since the last decade, with the increase in processing capacity, this technology has grown and been popularized. Although there is no consensus definition, it can be summarized as the ability of computers/processors to carry out operations or make decisions not based on preprogrammed orders but instead examples, the processing being learned from a set of data, through algorithms and complex calculations.13 So it is an evolving technology that encompasses several sectors, but its applicability in the health area stands out because, among other purposes, it helps to support the diagnosis, since it is based on looking for patterns and signs that can be difficult for the eyes of humans to recognize.14,15
Neural network - Machine learning - Deep learning: These are subclassifications of AI, but currently, they are common terms usually used separately. The term neural network represents different types of algorithms that aim to work in a similar way to the brain of an animal, being one of the bases of AI at the computational level.13 These algorithms are segmented into several classifications of their own, and among them, those most frequently applied in digital health are machine learning (ML) and deep learning (DL). ML refers to the concept where the “machine” is taught by example, that is, a database, usually text or numbers, being the most common form of AI. The DL differs from ML only because it learns to analyse much more complex information, such as image and video analysis.16,17
Big data: If AI technology acts on some type of data, it is evident that one of the elementary needs for its use would be the existence and storage of data. Big data is the broadest term in the topic, referring to the current existence of a huge amount of data. The wide diffusion of data, among other causes, is a result of the volume of data generated by the internet and the increase in storage capacity.18
Internet of Things (IoT)/Cloud computing: following in the wake of Big data, other terminologies deserve to be mentioned, which refer to ways of acquiring and storing data. The health area highlights the IoT, which refers to various devices that through biosensors they can collect data, providing information useful to monitoring and personalized health care. A classic example is the watches that measure heart rate and are able to generate information for users.19 To complete these definitions, the term cloud computing is used to refer to data that, after being collected, are stored on some physical server, not directly on the device but on a remote server, hence the term “cloud”. These same servers can also host applications, systems and even offer services related to artificial intelligence. It is also worth remembering that related to the collection and storage of data, issues related to the security and privacy of this information have been widely discussed today.20
Other terms: As already mentioned, the tools and technologies are in constant development, and their use and applicability are becoming more common and receiving greater prominence. Thus, in the health area, in addition to the previous terminologies, new terms such as virtual reality (systems that create a parallel digital reality21), augmented reality (systems that integrate the real world with digital components22) and blockchain (digital security technology used in banking/cryptocurrency systems) are being used with different applications in the health area (education, training or data protection).23
Digital health in vision sciencesAll of the main technologies surveyed are increasingly used during the development of digital health worldwide. Among the specialties that most explore this evolution is, without a doubt, eye care.10 The AI model for the diagnosis of diabetic retinopathy24 was a global highlight and still serves as an example in development in this area, with several examples worldwide (Healthtech sector of Google25 and others). Since then, the area of eye/visual health has become one of the richest in research and innovation, with investigations in different segments of digital health and with the use of different technological resources applied.26–30
In this sense, the present study aims to analyse the development of research in digital health applied to vision sciences to characterize its relationship with visual and eye health care, describing the technologies proposed and analysing the increase of these publication in time to update this information for health care researchers and practitioners.
MethodologyStudy designExploratory-quantitative research was carried out based on studies published in peer-reviewed journals, indexed and located in the SCOPUS database, regarding with the use of digital health technologies in vision care and research.
Data collectionFor the search, words directly related to the area of vision health were used, such as “ophthalmology”, “optometry”, “eye care” and “vision care”, combined with different terms and variations related to digital health technology, such as “ehealth”/”e-health”, “mhealth”/”m-health”, “big data”, “cloud computing”, “artificial intelligence”, “machine learning”, “deep learning”, “neural network”, “internet of things”, “augmented reality”, “virtual reality”, “blockchain”, “telemedicine” and “telehealth”.
The search for expressions was carried out with reference to the titles, abstracts and keywords of the studies, having as a complete initial search key: “(TITLE-ABS-KEY(optometry) OR TITLE-ABS-KEY(ophthalmology) OR TITLE-ABS-KEY("eye care") OR TITLE-ABS-KEY("vision care")) AND (TITLE-ABS-KEY(ehealth) OR TITLE-ABS-KEY("e-health") OR TITLE-ABS-KEY(mhealth) OR TITLE-ABS-KEY("m-health") OR TITLE-ABS-KEY("big data") OR TITLE-ABS-KEY("cloud computing") OR TITLE-ABS-KEY("artificial intelligence") OR TITLE-ABS-KEY("machine learning") OR TITLE-ABS-KEY("deep learning") OR TITLE-ABS-KEY("neural network") OR TITLE-ABS-KEY("internet of Things") OR TITLE-ABS-KEY("augmented reality") OR TITLE-ABS-KEY("virtual reality") OR TITLE-ABS-KEY(blockchain) OR TITLE-ABS-KEY(telemedicine) OR TITLE-ABS-KEY(telehealth))”.
Inclusion and exclusion criteria: The initial search was performed in January 2022, and 4488 records were found. Filters were applied directly to SCOPUS so that the research could be segmented according to the established inclusion criteria. Thus, for the search period, the last 10 years were defined from 2012 to 2021. Only articles published in scientific journals, in English and in a final stage of publication were considered. Journals within the thematic area of Medicine, Health Professions and Computer Science were included. After applying all of the criteria described, 1252 articles were chosen and analysed individually.
From these records, studies that were not directly related to the objective of the work (n = 30) and/or those that were not able to perform some form of categorization only by abstract, title or keywords (n = 153) were excluded from the detailed analysis. Thus, after applying the exclusion criteria, a total of 1069 articles were chosen for further analysis. The general scheme of the applied methodological procedure is summarized in Fig. 1.
Data analysisThe final records were analysed individually and through the information in the title, keywords and abstract to categorize each paper. The data were tabulated and analysed using Microsoft Excel/Access 365 and IBM SPSS Statistics 26.0 software.
For the categorizations adopted, in addition to a general descriptive analysis, the studies were classified into three large groups: regarding the technology used, regarding eye health, and finally regarding application use. A detailed schematic of the categorization process is provided in Fig. 2.
General descriptive analysisThe general characteristics of the analysed sample are presented, such as linked search words, counting of citations and countries involved in the study (according with authors' affiliation) providing a description of the number of reports published per year that describes the temporal evolution of the interest of these technologies within the sciences of vision in the last 10 years. To classify country involved in each publication we follow this rule: first; if more than one authors are affiliated to the same country, this country was counted just one time; second with authors of different countries, all involved countries were counted and third in case of authors with affiliations of different countries just the country of the main (first) affiliation was counted.
Regarding the technology usedAs different digital health tools are available, a detailed analysis of the main technology used or proposed in each report was conducted, classifying each result into four main categories, namely, eHealth, Big data, AI and Others. The members of the AI category were subclassified according to the type of technique used as ML or DL.
Regarding eye healthDifferent technologies in digital health have been proposed to assess different structures and/or eye disorders. Thus, these reports were divided into two main subgroups: eye structure and oculo-visual disorders.
The first (eye structure) indicates that they are specifically related to ocular structure assessment, which is classified into three categories according to the anatomical location: anterior eye, posterior eye and visual pathway. A detail analysis of the ocular structure was also conducted classifying results according the eye structure assessed. The second subgroup (oculo-visual disorders) includes the studies that are related to ocular pathologies and visual dysfunctions and is classified into four main categories: eye refraction and binocular vision, anterior eye, posterior eye and others. Also, a detail analysis of the eye condition or pathology assessed was conducted, classifying results according each assessed condition.
Regarding application useDigital health technologies allow for various applications in eye care and vision science, so the reports were classified according to their practical applications in two large blocks: clinical and no clinical applications.
Reports included in the clinical group involve studies with application of technologies related with different eye care procedure such as visual function (visual acuity, contrast sensitivity, visual field, etc.), anatomy (corneal topo/tomography, optic coherence tomography, etc.) and other measurements, where the purpose of technology can be applied in the practice of eye care practitioners and are classified into three main categories: clinical decision support, secondary/tertiary eyecare and primary eyecare. The No Clinical group included studies that had different purposes that were not directly related to clinical practice.
The specific topics identified, used for classification in each subgroup, was counted and presented to provide a more detailed view of the characteristics of the analysed studies.
It is necessary to clarify that all previously described categories and proposals of subclassification are not exclusive, which means that the same study can be included and categorized into different subgroups at the same time. For example, if an AI device (technology) that analyses the corneal topography (eye structure) is proposed to support clinical decisions (application/use) in cases of keratoconus (eye disorder), it will be classified and subclassified into different categories. However, when it was not possible to identify and define a specific classification for the study within a certain group, they were described as not identified.
Results and discussionGeneral descriptive analysisAmong the search results, 982 (91.86%) were directly related to the key term “ophthalmology”, while only 20 (1.87%) were associated with the term “optometry” (Table 1). The remaining 67 findings (6.27%) were related to different terms, such as “eye care”, “vision care” or their combination.
Additionally, there has been a systematic growth of research in this field over the last 10 years, as detailed in Fig. 3A. Within the analysed studies, a decade ago (in 2012), only 21 (1.96%) studies were found, while in 2021, the number of studies was 16 times greater, with 343 articles being found on this topic, which represents 32.09% of all analysed results.
Summary of the general data analysis (n = 1069) A) Representation of the scientific production of digital health technology applied to visual health from 2012 to 2021. Studies that also addressed the topic of the pandemic/COVID-19 (2020–21) are highlighted in black. B) List of the 15 countries with the highest number of publications, considering the countries of the affiliated institutions, of authors of the study.
Although the increase has been constant, notably, the vast majority of cases (n = 902; 84.38%) have been concentrated in the last 5 years (2017–2021). It is also worth mentioning that the years 2020–2021 alone represent more than half of the found results (n = 590; 55.19%). This increase could be partly explained by the impact of COVID-19 on scientific research that has force to introduce distance working in healthcare professions widely supported with health care technologies.31,32 However, studies directly related to the topic COVID-19 only represent 7.8% during these years (n = 46; 12 in 2020 and 34 in 2021).
A total of 883 articles received citations, with a median of 5 citations per study, with the largest having 2623 citations. Regarding the authors' countries, a total of 73 were identified with the information provided by affiliated institutions. The United States (n = 279; 18.58%), China (n = 239; 15.91%), India (n = 205; 13.65%), the United Kingdom (n = 87; 5.79%), and Singapore (n = 48; 3.20%) held the top 5 positions in the number of research papers, accounting for 57.12% of the results found (Fig. 3B).
Regarding the technology usedIt was possible to identify the technologies used in all of the analysed studies, and they presented at least two (average of 2.27) combinations of resources; that is, they combined different technologies. As an example, a study on smartphone applications for health support, which stores clinical records in the cloud and makes use of machine learning algorithms, will be classified equally in the subgroup of eHealth (app), Big data (data in the clouds) and in AI (machine learning), as long as these interactions are identified in the abstract.
According to the results presented in Table 2, individually, the use of "mHealth" (n = 651; 60.90%) and "Deep learning" (n = 616; 57.62%) stand out relative to the other results, which is explained by the growth of this technology in other health applications.33,34 On the other hand, “cloud computing” (n = 3; 0.28%) represents the lowest-used technology in vision sciences reports.
Summary of the technology used in vision sciences studies. The term “% total” refers to participation considering the total number of studies, and “% group” refers to participation within the subgroup.
After grouping the results into 4 major segments in relation to the resource used, AI was the most frequently applied technology (n = 831; 77.74%), followed by eHealth (n = 734; 68.66%), Big data (n = 147; 13.75%) and Others (n = 89; 8.33%). Within the group identified by “Others”, the most frequently found technology was “virtual reality” (n = 74; 6.92%), mainly related to its use in education tools and/or simulations of different procedures. The highlight of AI is also identified in studies applied in other segments of the health area.35
The massive difference in the study volume between 2012 and 2016 (n = 167;15.62%) and the last 5 years (2017–2021) stands out, concentrating 84.38% of the studies (n = 902), demonstrating with clarity the trend in the development of digital health studies related to vision sciences. For a detail analysis, when subdividing data from the last 5 years into 2017–19 (prepandemic) and 2020–21 (postpandemic), one can also observe the expansion of the number of studies, especially two groups, the eHealth and AI groups (Fig. 4A), possibly slightly influenced by the needs generated as a result of facing the world pandemic (COVID-19) related to remote support for health care, that could stimulate more applications of these technologies in eye care.36 As of 2017, there was an increase in references to deep learning (Fig. 4B), helping AI technology to lead among the studies analysed, probably impacted by the first large AI-powered diagnosis of diabetic retinopathy published in 2019, provoking from then on a wave in this area.25,37 Subsequently, 2020–21 follows the growth, evidencing the high development of digital health technology in the vision sciences.38,39
Within the references that used AI, 731 studies (87.96%) could be classified into the type of technique used, being segmented into two large groups: machine learning and deep learning, among which the latter also includes references to neural networks. Thus, deep learning appears in 74.12% (n = 616) of the studies identified relative to applied AI, while machine learning appears in close to a third of that, with 32.25% of findings (n = 268). It is worth remembering that both techniques can be used jointly. The results found can be explained by the large number of studies focusing on image analysis, such as retinography, in which deep learning is the most commonly used technique for complex analysis.34 Regarding the evolution of its use over the years, the use of machine learning and simpler AI techniques has been reduced, unlike what happened with more complex techniques, such as deep learning/neural networks.
Regarding eye healthThe studies were characterized according to their ocular structures/segments and eye problems were assessed, with joint mentions in many studies. More than 2/3 (n = 728; 68.10%) of the investigations related to technology in digital health and vision sciences were related to structures of the posterior segment, followed by the anterior (n = 116; 10.85%) and finally, structures related to visual pathway (n = 42; 3.93%), as illustrated in Fig. 5B. In the individual analysis, we observed that the cornea and the lens were studied as structures more specifically found within the anterior segment, with 41 (35.34%) and 39 (33.62%), respectively. Ganglion cells (n = 29; 69.05%), followed by photoreceptor cells (n = 17; 40.48), were the most frequently investigated in the visual pathway. However, the number of findings related to the retina was highlighted (n = 679), which were directly associated with 93.27% of all studies related to the posterior segment (Fig. 5A).
Regarding diseases or related eye disorders (Table 3), the results follow the same trend, with more than half (n = 606; 56.50%) of digital health technology studies for visual/eye health directed related to posterior segment diseases. The small reduction in the study data and the classification by the posterior segment can be explained by the fact that some themes have joint references, such as myopia, which were segmented for study purposes, within the group of refractive problems; however, many of these studies also make references to the retina structure.
Association of digital health technology with vision sciences regarding eye diseases and associated visual dysfunctions.
Anterior segment diseases impacted by cataract-associated studies led the second-position group (n = 116; 10.85%). Eye refraction and binocular vision had 74 findings and were present in 6.92% of all analysed studies. Finally, the group Others, which included diseases identified but that did not fit into the previous subgroups, amounted to 1.40%, with 15 studies found.
In the individual analysis, the eye refraction and binocular vision group was highlighted for myopia, with 36.49% (n = 27) of the studies in this category in the 6th position (2.53%) among the vision problems found in the sample. Strabismus, with 16 findings, followed by amblyopia (n = 12), was the most frequently found oculomotor and developmental disorder, with 21.62% and 16.22% participation in the subgroup. As already mentioned, cataract hoarded research in the anterior eye (n = 100; 81.21%) and was the 4th most frequent (9.35%) overall.
Again, in the posterior segment, the most common diseases in the studies were related to the retina, more precisely retinopathy, with 345 occurrences (57.11%), mostly diabetic retinopathy (n = 298; 49.34%). They accounted for almost 1/3 of the related studies in the area (32.30%). These results again strengthen the enormous impact of the first research related to the diagnosis of retinopathy using AI (deep learning), gaining prominence and being an example in the application of technology in the health area.24,34
It is worth remembering that many articles approach structures and ocular/visual problems together, as the same technological solution is often designed as an alternative for more than one problem, which explains the repetition in the references found. Nevertheless, in 271 (25.35%) of the analysed studies, it was not possible to identify the eye structure, and in 356 (33.30%), it was not possible to identify which eye problems were associated with the study, demonstrating the difficulty of data segmentation. However, this approach could be useful to provide a snapshot of the use of these technologies in eye health in the last decade.
Regarding application useThe different applications (clinical or not) of the results of the studies found are described in Table 4. The vast majority of studies in vision science associated with digital health technologies may have some form of clinical use (n = 1002; 93.73%), while nonclinical studies totalled 536 reports (50.14%) and in 24 studies, it was not possible to identify any form of classification.
Types of practical applicability of the studies evaluated.
In the general analysis of the clinical use group, the results show that most investigations looked for solutions to support decision-making (n = 952; 95.01%), followed by studies that referred to practices aimed at secondary and tertiary vision care, with 692 occurrences (69.06%), and finally, the studies related to activities aimed at primary vision care, with 15.27% (n = 153) participation. The large number of studies aimed at clinical practice, especially in decision-making, is expected to be a very strong line of research within AI to predict something and thus support the professionals in making decisions.40
Digital health technologies have been characterized by serving as support and assistance to professionals in their decision-making.41 Thus, the category decision-making support was subdivided into different types of decision support that the technology can support, that were identified in the studies of the evaluated sample, and that may also appear together. In this way, we realized that applications aimed at supporting a diagnosis (n = 506; 53.15%) was the most common association but it was closely followed by analysis tools (n = 479; 50.32%), classification (n = 328; 34.45%) and control (n = 301; 31.62%). It is worth noting that the subcategories were classified according to their mentions in the studies, but many ended up occurring together, so it is understandable why the term “prediction” appears with fewer references, as it is presented in a hidden way in studies that aimed at diagnosis, prognosis and management.
Within the subclassified group with use directed to the secondary and tertiary eye health care group, 225 reports of screening studies were found, representing 32.51% in this group. This activity was included in this subgroup when related to the screening of complex eye pathologies. These data were strengthened with the references most found in the sequence, including tomography and retinography (fundus eye), which found 212 (30.64%) and 187 (27.02%), respectively, suggesting once again that posterior structures are the most researched part to date.42,43
Regarding the subcategory of studies focused on procedures related to primary eye care, the main reference was visual acuity, with 103 linked studies (67.32% of this group). On the other hand, the mentions of contact lenses and glasses were the ones for which the least associated research was found, both with 4 findings (2.61%), drawing attention to the low number of studies in optometry and primary activities, in contrast to other groups.
Finally, in the no clinical practical use group, the general purposes of the studies were listed. Thus, the main highlights were processes related to Standardization (standards in identification and recognition of data) and Telemedicine, with 274 (51.21%) and 226 (42.16%) occurrences in the studies. For the first, they refer primarily to the search to know, dominate and establish reference standards for validations of AI algorithms, suggesting a process of evolution of the studies. Regarding the findings on telemedicine or telehealth, it is noteworthy that they had their greatest impact over the last two years (n = 110) during the pandemic, where the number of studies in this area was highlighted.44
Study limitations: The aim limitation of this review is related with the assessment of the information included in abstract, title and keywords that could not include with sufficient detail all details about how study was conducted and could affect to paper classifications if some information was not correctly identified or justify the relatively low number of papers identified in literature search. It is also possible that the manuscripts only include the description of the different types of algorithms, making their identification difficult. In addition, different papers could have different areas of study (health/computing) and use different terminology, making difficult compare or classify them.
ConclusionGiven the analysed information, the results of this report indicate that research in digital health applied to vision sciences has significantly increased in the last 5 years, with huge developments in AI, especially with the use of DL techniques applied to vision health. The most represented ocular structures in the studies are related to the posterior eye segment, with high interest in the retina, mainly because most common eye disorders are also related to this eye structure (for instance, diabetic retinopathy and AMD). Most studies are aimed at practical use and supporting clinical decision-making by vision health professionals, but with little presence in solutions and studies aimed at primary visual care.
Disclosure of conflicts of interestNone of the authors has a financial or proprietary interest in any material or method mentioned. Also, the authors declare that this research received no specific grant from any funding agency in the public, commercial, or not-for-profit sectors.
Delete footnote. This information has not interest for readers, it was just for Editor and Reviewers.