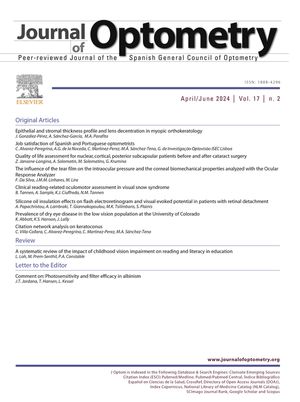
Artificial Intelligence, Data Science and E-health in Vision Research and Clinical Activity
More infoThe objective of this study is to analyse co-authorship and co-citation networks of publications in the field of artificial intelligence in ophthalmology and optometry. As well as, identify the different areas of research and the most cited publication.
MethodA search of publications was performed in the Web of Science database for the period from 1977 to December 2021, using the term “Artificial Intelligence AND (Ophthalmol* OR optometry)”. The analysis of the publication was carried out using the Citation Network Explorer, VOSviewer and CiteSpace software.
Results1086 publications and 2348 citation networks were found. 2020 was the year with the highest number of publications, a total of 351 publications and 115 citation networks. The most cited publication was “Clinically applicable deep learning for diagnosis and referral in retinal disease” published by De Fauw et al. in 2018, with a citation index of 723. Through the clustering function, three groups were found that cover the main research areas in this field: retinal pathology, anterior segment and glaucoma.
ConclusionsThe citation network analysis offers an in-depth analysis of scientific publications and the adoption of new topics and fields of research. The results of an exhaustive analysis of citation networks in artificial intelligence in the field of ophthalmology and optometry are presented since the publication of the first article in 1977.
Given the anticipated growth in the ageing population in the near future that will result in a higher rate of visual impairment and blindness, healthcare systems all around the world are making considerable efforts to improve eye-care.1
Nowadays, even in developed countries, the provision of ophthalmology consultations and care available is no longer sufficient if we take into account the increasing number of visually impaired patients.2 A study carried out in England found a permanent reduction in visual acuity and visual field in patients due to a 22-week delay in eye care. This could have been avoided if they were intervened earlier.3 This shows the urgent need for solutions to be implemented in order to improve the availability and accessibility of eye care services at primary, secondary, and tertiary level.
Medical imaging is fundamental for the proper diagnosis and treatment of ocular pathologies.4,5 In recent years, thanks to technological and therapeutic advances the resolution of these images, which can be used to provide information on anatomical and functional changes has significantly improved.6 Nevertheless, due to the high number of images and findings that can be obtained for each individual patient, these improvements have made actually made it more difficult to understand and treat eye diseases.7
Artificial intelligence (AI) can be used in clinical practice to help detect high-resolution image features, therefore reducing the incidence of diagnostic errors, and, as a result it is becoming an essential tool in ophthalmology. Given its ability to recognise specific disease patterns, this tool can be used to broaden scientific and innovative knowledge, and, likewise, it facilitates progress in the implementation of telemedicine detection programmes.8
Nowadays, AI is considered as human intelligence acquired by a machine or a computer through different means. It is comprised of machine learning (ML), deep learning (DL), conventional machine learning (CML), computer vision, robotics, reasoning, general intelligence, expert system, automated learning, and scheduling.9-11 Machine learning was first defined in 1988 as a “field of study that gives computers the ability to learn without being explicitly programmed, providing systems with the ability to automatically learn”,12 meaning therefore that it is constantly evolving. DL is another widely used concept, in which several levels of abstraction are involved in processing input data before this information is subsequently recognised through its projection in a lower dimensional manifold.13 The main difference between CML and DL is that, in the latter, the information is extracted and classified directly from the input, therefore allowing for a complete learning, while in CML, the input information is classified from hand-designed features.8 Therefore, the goal of AI is to get computers to provide information from our thoughts and improve work efficiency in today's fast-paced life.
In the field of ophthalmology, DL is being used to obtain images of the fundus of the eye, in optical coherence tomography and campimetry. It can be used to obtain a classification of the detection of DR, retinopathy of prematurity, optic nerve size, macular age, or age-related macular degeneration (AMD). DL can be used in teleophthalmology consultations to detect, diagnose and control ocular pathologies in patients within a primary care or community setting. However, a series of clinical and technical challenges prevail, which include the explanation of results, medical-legal issues, and its acceptance by physicians.14
This study presents a citation network analysis in which the relationship between the authors and publications was analysed. It also presents an in-depth analysis of the research areas considered to be of greatest interest within the research field of AI applied to ophthalmology and optometry.
MethodologyTo focus the search on articles on artificial intelligence in the field of optometry and ophthalmology, and avoid repetition of publications in the different searches, the Boolean operators NOT, AND and OR were used. In this way, the search terms used in the first search were: Artificial Intelligence AND (Ophthalmol* OR optometry), in the second search were “machine learning" AND (Ophthalmol* OR optometry) NOT Artificial Intelligence and in the third schear were “Deep learning” AND (Ophthalmol* OR optometry) NOT Artificial Intelligence NOT “machine learning".
The first articles on AI in the optometry and ophthalmology field were published in 1977, therefore the selected time interval was from 1977 to December 2021. The publications were searched and downloaded on the 18th of December 2021.
The Citation Network Explorer software was used to analyse the publications and visualise the citation networks. Using the citation score attribute, the most cited publications were quantitatively analysed. External connections were included that is, from other databases (Medline and KCI- Korean Journal database), and also Web of Science connections. These databases provide essential information for bibliometric and citation network analysis, including title/abstract, keywords, number of citations, and author affiliations, which may not be available in other databases.
The clustering function, using the formula developed by Van Eck in 2012, determines the publications that are most connected to each other. That is, this function is used to divide the publications into different groups based on the connections between them, so that the most connected articles are generally in the same group based on citation network criteria.15
The publications that are considered as the core of a citation network were analysed using the Identifying Core Publications functionality. Thus, publications that presented four or more citations in the citation network were included. This minimum number of connections is established by the research group, according to the criteria described in previous studies.15 Thus, those publications that have at least a connection with four other publications are selected. The drilling down functionality was used to analyse in depth each of the groups at different levels.
The scientometric analysis was performed using the CiteSpace software (5.6.R2). The H index was used to analyze the quality and level of academic output; This index is calculated by evaluating the number of citations of certain articles published in a journal: h of the N published articles have been cited at least h times; the degree was used to indicate the number of connections that exist among the authors, institutions, countries, and so on. That is, the number of times a country, an author or an institution is cited and by whom. In the case in which the degree value is higher, this suggests a greater level of communication and cooperation between the authors, institutions or countries. The centrality value was used to measure the importance of the nodes within the research cooperation network; and the half-life parameter was used to represent the continuity of institutional research from a time perspective. It is defined as the number of years that a publication receives half of its citations since it was published. A low dating half-life suggests dating activity that peaks and declines rapidly. A high cited half-life suggests dating activity that peaks and declines more slowly16,17
Results1138 publications and 2456 citation networks were found from all the fields in the search conducted in WoS.
The number of publications on this topic has increased significantly since 2018 (1977-2017: 13.3%; 2018-December´21:86.7%). 2020 was the year with the highest number of publications, accounting for 351 publications and 115 citation networks.
Description of the publicationsOf all the publications, 52.3% were articles, 18.4% were review articles, 12.2% were meeting abstracts, 9.9% were proceedings papers, 6.1% were editorial materials and the remaining 1.1% were letters to the editor, book chapters, corrections, additions or news items.
Language and countriesWith regards to the language of the publications, 97.8% were in English, 2.0% were in German, 0.1% were in Korean, and 0.1% were in Spanish. Therefore, the countries with the highest number of publications were the United States (36.7%), China (19.6%) and England (14.5%).
Authors and institutionsAs shown in Table 1, the authors with the highest number of publications on keratoconus were Daniel S W Ting (4.4%), Tien Yin Wong (3.9%) and Haotian Lin (3.3%).
Top 10 authors with the highest number of publications.
The institutions with the highest number of publications (Table 2) were the National University of Singapore (8.7%), the Singapore National Eye Centre (8.6%) and the University of London (8.5%).
Top 10 institutions with the highest number of publications.
Table 3 shows the main journals and the number of publications in each journal according to the WoS database.
Top 10 journals with the most publications.
Additionally, the most commonly used keywords were: deep learning (Frequency: 164; Degree: 25; Total Link Strength: 1152), machine learning (Frequency: 125; Degree: 29; Total Link Strength: 805) and diabetic retinopathy (Frequency: 73; Degree: 30; Total Link Strength: 1061). Figure 1 shows the most used keywords in the most relevant publications.
Most cited publicationsThe most cited publication was the article by De Fauw et al.,18 which was published in 2018, and which presented a citation index of 121. In this study, a new DL architecture was applied to a set of three-dimensional optical coherence tomography scans, segmenting a much larger range of tissue types relevant to the diagnosis of retinal pathologies and connecting said segmentation to relevant real-world referrals recommendations. This use of this tool will help eye care practitioners to refer patients. Likewise, this study also demonstrated that tissue segmentations are device-independent.
After analysing the 20 most cited articles, it was determined that 19 of them analysed AI systems for the diagnosis and prevention of retinal pathologies; and the remaining article analysed the detection of corneal ectasia (Table 4).
20 most cited publications.
The clustering function identified 4 groups, 3 of which presented a significant number of publications. However, the remaining group only represented 1.1% of the publications.
With regards to clustering parameters, a resolution value of 1.0 (default value in CiteNetExplorer software) was considered, and the minimal publication size for each group was 12.
The color of an article represents its topic, and the lines that connect the elements represent links.
- -
In Group 1, 460 publications and 1826 citations were found across the whole network. The most cited publication was the article by De Fauw et al.,18 which was published in 2018 in Nature Medicine, and which also ranked first among the 20 most cited publications. Therefore, the articles in this group analysed the use of strong diagnostic technology comprised of artificial learning-based DL systems for the automation of retinal pathology screening. Likewise, the clinical implementation of these systems, and the path that must be followed in order to ensure an accurate and appropriate diagnosis were also considered (Figure 2).
- -
In Group 2, 91 publications and 167 citations were found across the whole network. The most cited publication was the article by Ambrosio et al.,19 which was published in 2017 in the Journal of refractive surgery. This study presented the Tomographic and Biomechanical Index (TBI), which combines Scheimpflug-based corneal tomographic and biomechanical data to improve the detection of ectasia. To that end, they retrospectively analysed 72 eyes with ectasia in patients from different continents who had not undergone a surgical procedure. The results showed that the TBI can be used to detect subclinical ectasia and confirm unilateral ectasia.
Therefore, the articles in this group described the uses of AI in the anterior segment, which covers keratoconus, corneal transplants, cataracts in adults and children, angle-closure glaucoma, and iris tumours. Moreover, they reviewed the achievements of machine learning at an ocular level (Figure 3).
- -
In Group 3, 61 publications and 139 citations were found across the whole network. The most cited publication was the article by Wang et al.,20 which was published in 2019 in Investigative Ophthalmology & Visual Science. In this study, by analysing changes in the spatial pattern, the authors detected the progression of the visual field. 12,217 eyes were selected that had at least five reliable 24-2 visual fields, in a period of 5 years with follow-up intervals of 6 months. The visual fields were decomposed into 16 archetype patterns previously derived by AI techniques, and it was determined that this method can be used to obtain information about visual field progression patterns.
Therefore, the articles in this group analysed current AI based DL methods to help eye care professionals understand their potential impact in the detection of glaucoma (Figure 4).
Core function413 publications with 4 or more citations were found across the whole network, which was comprised of 2456 citations, therefore representing 31%. This means that the research area is diverse. Nonetheless there is a clear focus on the research that is being carried out on this field, with the most commonly discussed topic being the use of AI in the diagnosis of retinal pathologies.
DiscussionThis study enabled us to obtain a comprehensive analysis of the available literature on the implementation of AI in the fields of ophthalmology and optometry. For this purpose, we chose to conduct a bibliographic search in the Web of Science database, given that its search range dates back to 1900. However, this database only considers journals of international relevance that have gone through a rigorous selection process.
The CitNetExplorer and CiteSpace software allowed us to determine the connections that exist between the different research fields and groups. By using the clustering function, we were able to group publications according to the relationships that exist between the citations. The drilling down function allowed us to conduct a more in-depth analysis of the bibliography for each group. Additionally, the core publications function helped us to identify the key publications for each group. Finally, scientometric analysis was used to obtain an important quantitative analysis of the existing literature to improve the understanding of this fast-growing field of knowledge.
The number of publications on this topic has increased considerably since 2018, while improvements in computer technology and large data sets over the last 5 to 10 years have allowed for the development of deep learning. These new advances have demonstrated that AI outperforms human performance in many fields of medicine and healthcare.14 The most cited publication, which was published in said year, was the article by Faw et al.18 Another relevant paper was that of Abramoff et al.,21 which was also among the 20 most cited publications. In this study, the authors found that the software developed for diagnosing DR outperformed all tests with a sensitivity of 87.2% (95% CI, 81.8% -91.2%) (> 85%), a specificity of 90.7% (95% CI, 88.3% -92.7%) (> 82.5%) and a resolution rate of 96.1% (95% CI, 94.6-97.3%). These findings led the Food and Drug Administration (FDA) to authorise the use of this software by healthcare providers for DR diagnosis and screening.
2020 was considered a key year due to the large number of publications. In that year, the publication by Milea et al.22 attracted particular attention. They discovered an AI and DL algorithm that could be used to perform retinographies. This algorithm was highly sensitive to detecting papillary oedema and normal optical nerves. However, negative predictive values were high, but the positive predictive values varied depending on the prevalence of papilledema in the study population. Another study was the one by Yim et al.,23 in which they developed an AI system, which is capable of predicting the risk of developing exudative macular degeneration in both eyes in patients who are already suffering from exudative macular degeneration in one eye. This system makes it possible to overcome the variability of the diagnosis among different experts, therefore obtaining better results.
With regards to the countries with the highest number of publications, our results were consistent with the findings of the bibliometric study by Wang et al.,24 as it was determined that the United States, China and England have the highest publication rates. However, in terms of the most published authors, only Michael F. Chiang appeared in both studies. It was the same in terms of the institutions, with Sun Yat-sen University and Johns Hopkins University appearing in both studies. This difference confirms the strong interest of researchers in this field, and the fact that bibliometric data varies over a short period of time. If we analyse the data from the two studies, we can conclude that the high publication rate in the United States is due to the fact that approximately 12 million people aged 40 years and older in the United States are visually impaired. This includes 1 million people who are blind, 3 million people who have a visual impairment after correction, and 8 million people who have a visual impairment as a result of an uncorrected refractive error. In addition, 6.8% of children under the age of 18 in the United States have been diagnosed with eye and visual disorders. Nearly 3% of children under 18 years of age are blind or have vision problems.25
The field of research which has attracted the most interest is the application of AI in the case of retinal disorders. It is estimated that by 2040, 600 million people worldwide will have diabetes, including 1/3 who will have DR.26 And about 288 million patients will suffer from AMD.27 Therefore, AI systems are being developed to improve the diagnosis and treatment of said conditions. Recently, Gulshan et al.28 created a DL system with excellent performance for the diagnosis of DR, which was developed using 128,187 retinal images, classified between 3 and 7 times for the diagnosis of DR and diabetic macular oedema by a group of 54 ophthalmologists and ophthalmology residents. The sensitivity and specificity were 87% and 98.5%, respectively. Subsequently, Ting et al.29 developed another system using the Singapore Integrated Diabetic Retinopathy Programme over a 5-year period with a selected dataset from 6 different countries (Singapore, China, Hong Kong, Mexico, United States, and Australia). In this programme the sensitivity and specificity were 90.5% and 91.6% respectively. All of these systems have worked well with the available data sets, nonetheless, none of them have tested for detecting DR in the real world. At the same time, the possibility of using DL systems in populations of different ethnic groups with retinographies captured using different cameras remains uncertain. Up to now, only the system developed by Abramoff et al.21 has been endorsed by the FDA. This software, called IDx-DR, consists of a non-hydrostatic retinal camera, capable of providing the following two results: 1. If more than mild DR detected, refer to an eyecare professional ECP; 2. If the results are negative for more than mild DR, rescreen in 12 months. For the diagnosis of AMD, the use of AI as diagnostic support for AMD treatment decisions is currently being studied. Thus, Prahs et al.30 analysed the manner in which AI is used to decide whether intravitreal injections are recommended for a patient, and it was observed that convolutional based neural networks can predict the need for anti-VEGF therapy in 95% of cases. The second leading area of research is AI in glaucoma. Thus, Li et al.31 and Ting et al.29 developed computer algorithms to detect a disk size consistent with glaucoma, defined as a vertical cup-disc ratio of 0.7 and 0.8 respectively. The researchers also applied ML methods to detect glaucomatous damage to the nerve fibre layer from normal wide-angle (9 × 12mm) OCT scans.32 In terms of the size of the visual field, Elze et al.33 developed a computer programme that identifies relevant visual field loss models and assigns each of them a weighting factor. This method has proven useful in detecting early visual field loss associated with glaucoma. A further study by Kazemian et al.34 developed a tool that uses tonometry and visual data to predict disease progression in different target IOPs.
The third area of research is the use of AI for corneal disorders. AI systems are currently being developed to prevent keratoconus and the risk of developing subclinical ectasia following refractive surgery. AI models can predict early graft dislocation using a larger residual interface fluid after refractive surgery.35
In conclusion, this study provided a comprehensive analysis of key research areas in the field of AI applied to optometry and ophthalmology. Thus, the topics where artificial intelligence is most used at that time and which have been the same since 1977 are analyzed.. The use of AI in the diagnosis of keratoconus and corneal ectasia remains an understudied and growing field. Since 2018 there has been a significant increase in the number of studies, thanks to advances in computer systems. AI will help improve the diagnosis and treatment of eye diseases and ophthalmological care in hospitals and primary care facilities.
Source of fundingNone.